Exploring Top Statistical Software for Business Insights
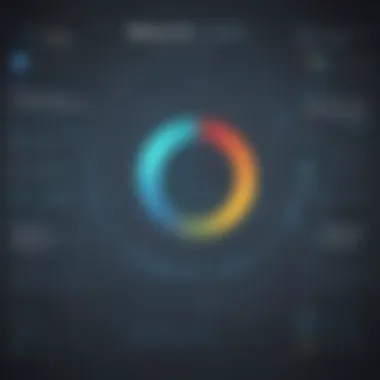
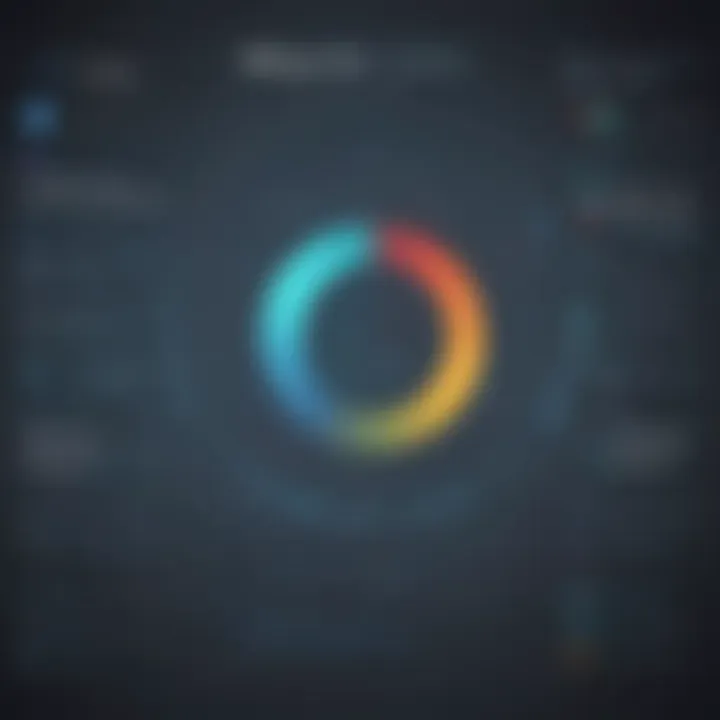
Intro
In today's data-driven world, statistical software plays a crucial role in processing and analyzing information. For small to medium-sized businesses, entrepreneurs, and IT professionals, selecting the right software can directly influence operational efficiency and strategic decision-making. This overview aims to guide readers through various statistical software options, emphasizing their core features, advantages, and limitations.
Understanding these tools enables one to make informed choices that drive productivity and foster business growth. Therefore, we will analyze not only the functionalities offered by these platforms but also their integrations with other tools, highlighting how they can work seamlessly within existing workflows.
Functionality
Statistical software varies widely in terms of core features. While each platform has distinct capabilities, this section outlines the most common elements found in popular software solutions:
Core Features Overview
- Data Management: The ability to handle large datasets, allowing users to import, clean, and manipulate data efficiently.
- Statistical Analysis: Tools for performing a variety of statistical tests, including t-tests, ANOVA, regression analysis, and more.
- Visualization: Features that enable users to create charts, graphs, and dashboards for better data representation and insights.
- User Interface: A focus on user-friendly designs can make a significant difference in user adoption and productivity.
Among these features, data management stands out as essential. Business owners need reliable ways to organize and interpret data to extract actionable insights.
Unique Selling Points
Each software has its distinctive features that set it apart:
- R: An open-source environment favored for its extensive packages and flexibility in statistical modeling.
- SAS: Renowned for its advanced analytics capabilities and strong support for data manipulation. Highly regarded in the healthcare and academic sectors.
- SPSS: User-friendly and widely used for social science research, offering robust tools for statistical analysis without requiring extensive programming skills.
Selecting the right software depends significantly on the specific needs of the organization and the ability of users to leverage these unique strengths effectively.
Integrations
The capability to integrate with other tools is vital for maximizing the potential of statistical software.
Compatible Tools
Compatibility can enhance workflows and data transfer between applications. Some commonly integrated tools include:
- Microsoft Excel: Almost all statistical software can import or export data from Excel, making it convenient for users already familiar with spreadsheets.
- Python and R: Many platforms support scripting for more advanced statistical computations, allowing users to extend functionalities.
- Power BI: For businesses focused on data visualization, integrating with Power BI can enhance reporting capabilities.
API Availability
Having a robust API is essential for organizations looking to automate their workflows. This feature allows developers to write custom functions or automate recurring tasks, delivering more value.
Many software providers, such as SAS and R, offer well-documented APIs, making integration seamless.
Choosing the right statistical software not only entails evaluating features but also considering how they fit into the existing technological landscape of your business.
Through this exploration of functionalities and integration capabilities, we have laid the groundwork for understanding the landscape of statistical software. In the following sections, we will delve deeper into specific tools and their practical applications in various fields.
Prologue to Statistical Software
Statistical software plays a crucial role in modern data analysis. These tools simplify the complex process of examining and interpreting data sets. They provide various functionalities that enable users to derive meaningful insights from their data. In todayβs data-driven world, understanding statistical software is essential for effective decision-making.
Definition and Purpose
Statistical software refers to computer programs designed for statistical analysis. These applications allow users to store, analyze, and visualize data in various formats. Their primary purpose is to assist in performing statistical tests and modeling, which are essential for making informed choices based on data.
Some common features of statistical software include data management, statistical analysis capabilities, and graphical representation of data. These tools can handle everything from basic calculations to advanced predictive modeling. As such, their use spans various fields, including business, scientific research, and social sciences.
Importance in Data Analysis
The importance of statistical software in data analysis cannot be overstated. As organizations accumulate vast amounts of data, the need for efficient analysis tools becomes paramount. Statistical software enables users to quickly process and analyze large data sets without manual intervention.
"Without proper tools, the data collected could remain misunderstood or underutilized."
Some critical benefits include:
- Efficiency: Statistical software speeds up the analytical process, allowing for quicker insights.
- Accuracy: The software minimizes errors that might occur in manual calculations, enhancing reliability.
- Visualization: Many statistical tools offer visualization options, which help to present data in clear and understandable formats.
Using statistics effectively empowers businesses to optimize operations, predict trends, and understand customer behavior. Thus, mastering statistical software is necessary for professionals involved in data-driven decision-making.
Overview of Statistical Software Types
The landscape of statistical software is diverse, encompassing both open source and proprietary options. Understanding the difference between these types is crucial for businesses and individuals seeking the best tools for their data analysis needs. Each type offers distinct advantages and comes with varying considerations regarding cost, ease of use, and support.
Choosing the right statistical software can influence the efficacy of data analysis significantly. Open source software often candidates for budget-conscious users, allowing them to leverage powerful tools without the constraints of licensing fees. Conversely, proprietary software may provide more polished interfaces and customer support, which can be crucial for organizations that require reliable assistance.
Open Source Software
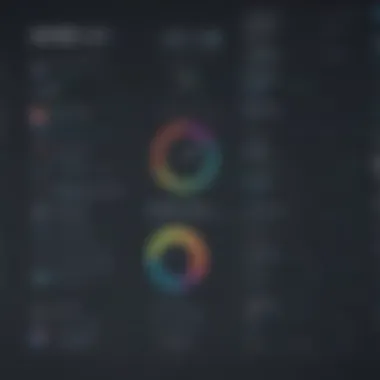
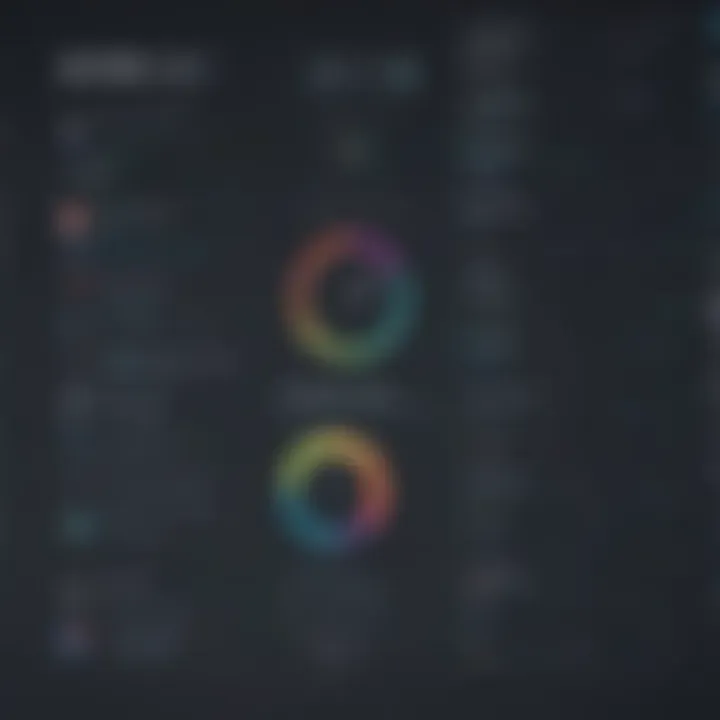
Open source statistical software, such as R and Python, allows users free access to its source code. This versatility enables customization, meaning users can modify the software to better meet their specific analytical needs.
Benefits of Open Source Software:
- Cost-Effective: Users do not need to pay for licenses, which is particularly beneficial for startups and small enterprises.
- Community Support: A strong community of users exists to offer support and contribute to the software's development. Furthermore, many resources for learning are available online.
- Flexibility: Users can adapt the software to their workflows. For instance, Rβs package ecosystem allows for extensive statistical methods.
However, open source software may pose some challenges. The learning curve can be steep for those without a programming background. Additionally, users might need programming skills to manipulate the software fully, which could limit its usage among those without a technical background.
Proprietary Software
On the other side, proprietary statistical software, such as SPSS and SAS, is often seen as more user-friendly. These tools typically come with comprehensive customer support and training resources to assist users.
Advantages of Proprietary Software:
- User-Friendly Interface: Most proprietary software packages offer intuitive interfaces that simplify the data analysis process for users.
- Comprehensive Support: Vendors provide robust customer service and training packages, which can lead to quicker onboarding for new users.
- Reliability: Proprietary solutions are often rigorously tested, providing assurances regarding performance and accuracy.
While advantages exist, there are downsides. Proprietary software typically requires a substantial financial investment, which can be a barrier for smaller organizations. Additionally, users may encounter limitations in customization compared to open source alternatives.
In summary, both open source and proprietary statistical software have unique strengths and weaknesses. A thorough assessment of oneβs specific needs and resources is essential before selecting software for statistical analysis. Understanding these types lays the groundwork for discussing individual software solutions in more detail.
Popular Statistical Software Solutions
In today's data-driven landscape, selecting the right statistical software is crucial for effective decision-making. The Popular Statistical Software Solutions section is essential as it narrows down a myriad of options into a few significant players. Understanding each software's core strengths illuminates paths in data analysis, research, and business strategy implementation. Statistical software is more than just tools; they are enablers of insights that can drive innovation and efficiency.
R
R is a powerful software environment primarily used for statistical computing and graphics. As an open-source platform, it attracts a community of enthusiasts who contribute to an extensive range of packages. This flexibility allows users to conduct various analyses, from basic descriptive statistics to complex modeling. One of R's greatest strengths is its visualization capabilities. Users can create intricate plots that reveal patterns and trends in data effectively. Furthermore, R maintains compatibility with other programming languages, enhancing its functionality and adaptability in diverse analytical environments. Business analysts considering R should evaluate the learning curve, as it may appear intimidating initially for novice users.
Python with Statistical Libraries
SPSS
SPSS, or Statistical Package for the Social Sciences, is a widely used proprietary software for statistical analysis. It is particularly favored in social sciences and market research. SPSS is recognized for its user-friendly interface, which facilitates quick data analysis without extensive programming knowledge. The software comes equipped with a variety of statistical tests and analyses, including regression, ANOVA, and descriptive statistics. Its ability to handle large datasets makes it an option for organizations dealing with voluminous information. However, as a proprietary solution, licensing costs may deter some smaller businesses.
SAS
SAS is a comprehensive software suite used for advanced analytics, business intelligence, and data management. Its strengths lie in its robustness and flexibility, making it suitable for industries like financial services and healthcare. SAS provides a high level of support and documentation, helping users navigate complex analytical tasks. The software offers features for data manipulation, statistical analysis, and predictive modeling. Despite these advantages, SAS can be expensive for smaller operations. Hence, business entities must weigh the cost against the potential analytical capabilities it provides.
Stata
Stata is another renowned statistical software that finds favor among researchers in economics, sociology, and political science. Its distinct advantage is the balance it strikes between user-friendliness and a wide array of statistical functions. Stata offers powerful data management, visualization, and statistical testing capabilities, making it suitable for longitudinal data analysis and complex survey data. The software continually updates its features, ensuring it remains current with user needs. Organizations considering Stata must account for its price, typically more affordable than SAS but still significant for some.
In summary, the choice of statistical software is pivotal in todayβs analytical landscapes. Understanding various software solutions ensures organizations make informed decisions aligned with their data needs and budget.
Key Features to Consider
When selecting statistical software, understanding the key features is crucial. Various functionalities can significantly affect usability and productivity for small to medium-sized businesses, entrepreneurs, and IT professionals. Highlighting these features can inform better choices that align with specific needs.
User Interface
A software's user interface (UI) plays an essential role in its adoption and daily use. An intuitive UI allows users to navigate the software more effectively. For example, drag-and-drop functionalities and clearly labeled menus can enhance user experience. A confusing interface may lead to frustration and errors, especially for those who may not be tech-savvy. It is beneficial to consider whether the software offers a customizable dashboard that can adapt to different tasks. Furthermore, built-in tutorials and comprehensive documentation can aid in reducing the learning curve.
Data Handling Capabilities
Robust data handling capabilities are fundamental for any statistical software. This includes the ability to support various data formats, such as CSV, Excel, and SQL databases. The capacity to manage large datasets without significant lag should also be evaluated. Furthermore, data cleaning and transformation features are vital for preparing data for analysis. Efficient data handling can save time and minimize errors, which is particularly important for decision-makers who rely on accurate data. Effective integration with other data sources, such as APIs, is also a key consideration. This can streamline workflows and enhance analytical capabilities.
Statistical Techniques Offered
The breadth of statistical techniques available in the software is another crucial feature. Basic descriptive statistics are often insufficient for comprehensive analysis. Advanced techniques, such as regression analysis, hypothesis testing, and machine learning algorithms, are vital for extracting actionable insights. Thus, one should evaluate if the software aligns with the complexity of the analysis required. Moreover, robust simulation and modeling capabilities can provide deeper understanding of potential outcomes. Itβs beneficial to check both the depth and variety of options available to ensure they meet the requirements of different projects.
"Choosing the right statistical software can greatly enhance your analytical capabilities and decision-making processes."
In summary, when evaluating statistical software, the user interface, data handling capabilities, and range of statistical techniques offered should be prioritized. These elements can directly influence productivity and the accuracy of insights derived from data analysis.
Comparative Analysis of Top Software
The comparative analysis of top statistical software is essential in understanding the strengths and weaknesses of each tool. With numerous options available, businesses and researchers must determine which software aligns best with their specific needs. This analysis not only identifies key features and functionalities but also presents insights into how these tools perform in practical scenarios. This section aims to highlight differences and similarities among leading software, providing clarity for users navigating a complex software landscape.
R vs Python
R and Python are both powerful programming languages commonly used for statistical analysis. R excels in statistical computation and graphical representation. Its extensive library of packages offers a range of functions for specific statistical needs. Some notable packages include , , and . This makes R particularly appealing for statisticians and data miners.
In contrast, Python is a versatile language that supports multiple programming paradigms. With libraries such as , , and , Python provides a broader scope of data manipulation and analysis. The choice between R and Python often boils down to the userβs background; R is preferred by statisticians, while Python is favored by software developers and data engineers.
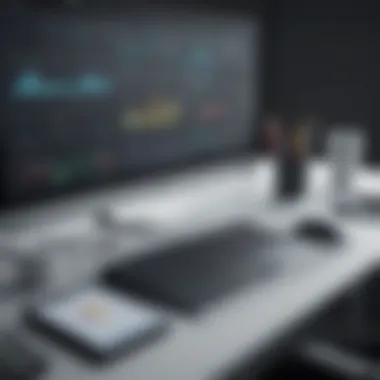
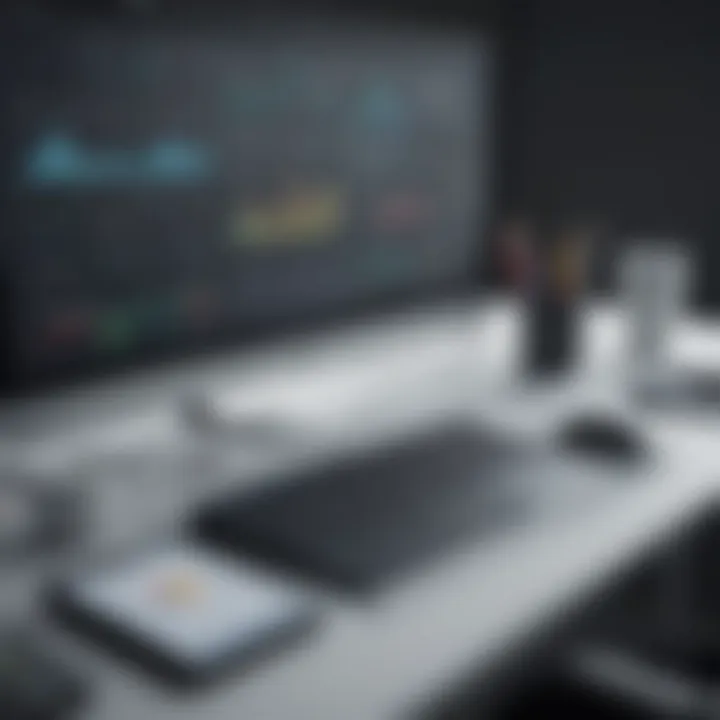
"Choosing between R and Python ultimately depends on the specific project requirements and the user's proficiency with the language."
SPSS vs SAS
SPSS and SAS are two stalwarts in the realm of statistical software. SPSS, developed by IBM, is widely used in social sciences for data analysis. Its user-friendly interface allows users to perform complex analyses without extensive programming knowledge. Common applications include survey data and psychological studies.
SAS, on the other hand, is a more robust solution favored in enterprise environments. It excels in data management and predictive analytics. SAS requires a steeper learning curve compared to SPSS, but it offers extensive documentation and support for advanced data analysis, specifically in sectors like healthcare and finance.
Key differences include:
- Usability: SPSS is easier for beginners.
- Capabilities: SAS has more advanced analytics options.
Stata in Context
Stata serves a niche but significant role in the realm of statistical software. Primarily utilized in academic research and policy analysis, it stands out for its strong data management capabilities and efficient handling of large datasets. Stata offers a balance between ease of use and advanced statistical techniques.
The software is particularly recognized for its commands tailored for econometric analyses and surveys. Researchers often choose Stata for its speed and reliability when conducting complex statistical tests. Furthermore, the extensive documentation and active user community enhance the overall user experience.
In summary, while R, Python, SPSS, SAS, and Stata have unique advantages, the choice should be predicated on the particular use case. Each has its place in the statistical landscape, serving varied functions across different industries.
Licensing and Pricing Models
In the realm of statistical software, understanding licensing and pricing models plays a crucial role in the decision-making process for businesses and individuals alike. The right model can influence user accessibility, budget constraints, and overall return on investment. As organizations delve into statistical tools, the financial implications of these choices cannot be overlooked. This section unpacks the two primary models prevalent in this domain: free software options and subscription-based pricing.
Free Software Options
Free software options provide several advantages, notably accessibility and a lower barrier to entry. Users can explore and utilize powerful statistical tools without incurring heavy costs. Notable examples include R and Python, which offer robust libraries for statistical analysis.
Utilizing free software can be a game-changer for small to medium-sized businesses and startups. These platforms often have vibrant communities offering extensive documentation and support.
However, it is essential to consider the trade-offs. Free software can sometimes lack comprehensive user support compared to paid alternatives. Additionally, users may experience a steeper learning curve, requiring more time to gain proficiency. Despite these challenges, the benefits of cost savings and flexibility are compelling for many users.
Subscription-Based Pricing
Subscription-based pricing represents a more structured approach to accessing statistical software. This model usually entails a monthly or annual fee for usage. Well-known platforms, such as SPSS and SAS, primarily operate under this model.
One significant benefit of subscription-based software is the inclusion of regular updates and customer support. Users receive the latest features and security enhancements without needing to manually upgrade their systems. This can be crucial for businesses that rely heavily on data analysis.
On the financial side, subscription models can sometimes lead to higher overall costs in the long run, especially for long-term users. Therefore, evaluating the overall budget and long-term needs is necessary before committing.
Ultimately, the model chosen should align with the organizationβs size, budget constraints, and specific analytical needs. Understanding these options empowers decision-makers to select platforms that best fit their strategic goals and resources.
Integration with Other Tools
In the modern landscape of data analysis, the ability to effectively integrate statistical software with other tools cannot be overstated. This integration plays a crucial role for businesses. It enables a seamless workflow, enhances data versatility, and amplifies the analytical capabilities of users. The interconnectedness of these tools allows for more powerful data insights, which is vital for informed decision-making in todayβs competitive environment.
Data Visualization Tools
One of the foremost elements of integration is with data visualization tools. Software such as Tableau and Microsoft Power BI can connect with statistical programs like R and Python. This connectivity allows users to transform complex statistical outputs into visual representations. Such visualizations help in grasping the data intuitively. They cater to various audiences by simplifying intricate findings into digestible graphics.
- Enhanced Understanding: Charts and graphs translate numbers into visuals, making interpretation easier for stakeholders who may not have a technical background.
- Customizable Reports: Visual tools often provide options for tailoring reports to suit specific needs, which can be pivotal in meetings or presentations.
- Enhanced Decision-Making: With clear visualizations at hand, teams can make quicker decisions as they can easily spot trends and patterns.
Integrating statistical software with data visualization tools empowers businesses to leverage the full potential of their data. It not only increases the effectiveness of data analysis but also streamlines the communication of findings.
Database Management Systems
Another critical integration aspect involves database management systems, such as Oracle, MySQL, and Microsoft SQL Server. Many statistical software solutions can directly access data from these systems. Such access is valuable for various reasons:
- Efficient Data Retrieval: Users can directly pull large datasets into their statistical software, reducing the time spent on manual imports.
- Real-Time Analysis: Integration permits the analysis of live data, which is vital for businesses that rely on up-to-date information for their operations.
- Data Integrity: By accessing the data directly from a database, the consistency and reliability of that data is generally higher compared to manual uploads.
Overall, the synergy between statistical software and database management systems fosters an environment where analysts can focus on interpreting data rather than the mechanical aspects of data handling.
Integrated tools create a more efficient workflow, allowing users to gain insights faster and with greater accuracy.
Real-World Applications of Statistical Software
Statistical software plays a significant role in various industries and sectors, influencing how data is analyzed and decisions are made. The applications of these tools extend beyond theoretical frameworks, illuminating practical routes to harnessing data effectively.
Statistical software can greatly enhance operational efficiency, guiding critical decisions based on empirical evidence rather than intuition alone. This reliability allows for deeper insights into trends and patterns, serving as a catalyst for innovation and development.
Research and Academia
In research settings, statistical software is indispensable for data analysis, hypothesis testing, and interpreting results. Researchers utilize tools like R and SPSS to process large volumes of data, applying diverse statistical methods to validate their studies.
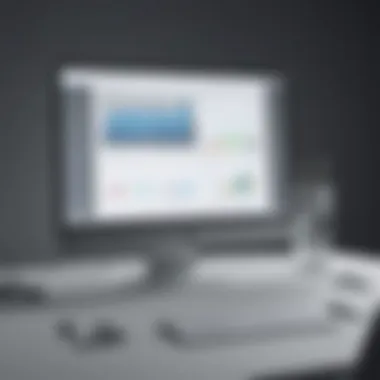
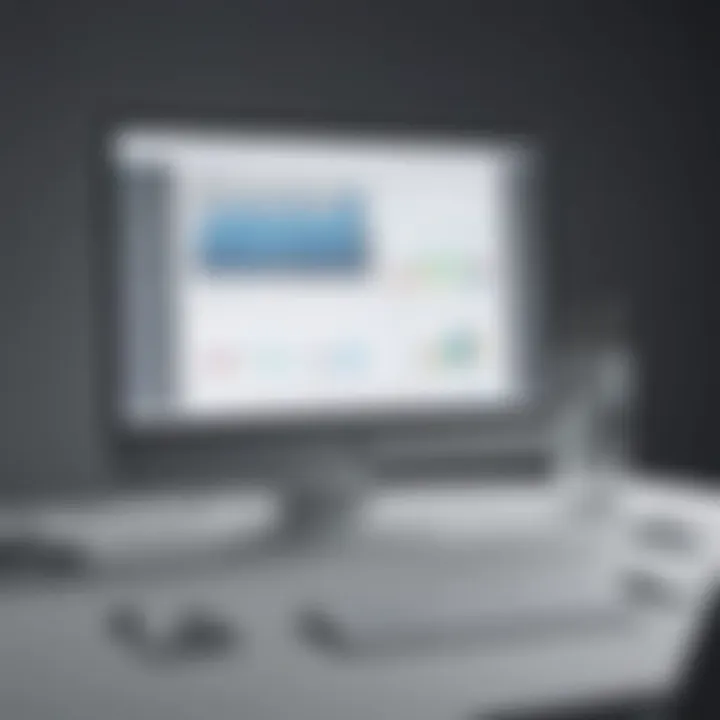
The capabilities of these software solutions facilitate:
- Data Management: They simplify the tasks of cleaning, organizing, and preparing data for analysis, enabling researchers to focus on meaningful insights.
- Advanced Analysis Techniques: From regression analysis to factor analysis, these tools support various statistical methods, catering to diverse research needs.
- Reporting and Visualization: A clear presentation of findings is essential. Software often includes functionalities for creating graphs and charts that make complex data comprehensible.
Moreover, the reproducibility of research is enhanced when proper statistical methods and software tools are used, fostering transparency and credibility across academic fields. Collaboration on projects is also smoother, as researchers can share scripts and results generated from the same software platform.
Business Analytics
In the realm of business, the application of statistical software translates into informed decision-making that drives growth and efficiency. Companies leverage software like SAS and Stata for analyzing market trends, customer behavior, and operational performance.
The significance of statistical software in business lies in:
- Predictive Analytics: Businesses can forecast future trends and behaviors by analyzing past data, allowing for strategic planning and resource allocation.
- Performance Metrics: Statistical analysis helps organizations evaluate business performance against key indicators, facilitating better management of resources and personnel.
- Market Research: Understanding customer preferences and market dynamics becomes clearer when employing statistical methods. Businesses use survey analysis tools to capture consumer insights, guiding marketing strategies.
Moreover, small and medium-sized enterprises can greatly benefit from statistical software by identifying inefficiencies and deriving insights that would otherwise remain hidden. This level of data-driven analysis helps businesses not only survive but thrive in competitive markets.
"Statistical tools empower businesses to analyze data competently, informing strategic choices that can lead to increased efficiency and profitability."
Challenges in Statistical Software Usage
Understanding the challenges associated with statistical software is crucial for businesses aiming to leverage data effectively. While statistical software offers numerous benefits, such as enhanced data analysis capabilities and improved decision-making, it also presents specific difficulties that users must navigate. Recognizing these challenges allows users to develop strategies to mitigate them, ensuring more effective use of their software choices.
Learning Curve
The learning curve is one of the most significant challenges users face when adopting new statistical software. Each program has its own interface and set of features, which can be daunting for beginners. Depending on the complexity of the software, new users may find themselves spending substantial time learning how to utilize the full potential of the tool.
- Training resources and support networks can help users overcome this barrier. Many software vendors offer tutorials, webinars, and community forums where users can share experiences and seek guidance. However, the availability and quality of these resources may vary, affecting user experience and satisfaction.
- In small to medium-sized businesses, the speed at which teams can adapt to new software is crucial. A prolonged adjustment period can defer project timelines and reduce productivity.
- Consideration for Time Investment: To improve the learning experience, businesses should allocate sufficient time for employees to engage with training materials. This may involve scheduling dedicated training sessions or providing access to online courses.
By addressing the learning curve effectively, companies can better ensure that their teams are proficient and confident in using statistical software, ultimately leading to more insightful analysis and data-driven decisions.
Data Security Issues
Data security is another pressing concern. As organizations increasingly depend on software to analyze and manage sensitive data, ensuring privacy and protection becomes paramount. Statistical software may handle personal information, financial data, or proprietary business insights, all of which are susceptible to breaches if not managed properly.
- Users must be wary of how data is stored and processed. Not all statistical software platforms adhere to strict security protocols, which can leave vulnerable gaps.
- Frequent updates and patches are critical. Software that does not regularly update may expose users to risks as new threats emerge. Organizations should prioritize tools that demonstrate a commitment to data security and provide transparent, detailed information about their security measures.
- Regulatory Compliance: In many industries, adherence to regulations such as GDPR or HIPAA is mandatory. Choosing software that aligns with such requirements is essential. Companies should conduct thorough research to ensure their chosen tools meet legal standards and best practices.
Future Trends in Statistical Software
As businesses and organizations increasingly rely on data-driven decision-making, understanding the future trends in statistical software becomes crucial for adapting to innovations and optimizing analytical capabilities. With technological advancements, statistical software is evolving at an unprecedented pace. This section will explore two significant trends: the integration of artificial intelligence (AI) and machine learning (ML), and the shift towards cloud-based solutions. Each trend holds particular importance for various domains, affecting the way statistical analyses are conducted.
Emergence of AI and Integration
The integration of AI and ML into statistical software is reshaping the landscape of data analysis. These advanced technologies allow statistical packages to handle more complex data sets and to apply predictive analytics effectively. For small to medium-sized businesses, this means accessing tools that can uncover insights previously unattainable with standard statistical methods.
Using AI and ML, statistical software can automate routine tasks. This reduction in manual effort not only saves time but also minimizes human errors in analysis. Furthermore, algorithms can learn from previous datasets and improve their predictive accuracy over time. This capability enables businesses to make more informed decisions based on real-time data analysis.
Moreover, AI-driven tools can assist in identifying patterns and trends in data that may not be immediately obvious. This can lead to enhanced strategic planning and innovation. For example, a retail business can utilize AI algorithms to analyze customer buying behaviors, allowing for better inventory management and tailored marketing strategies.
The integration of AI and machine learning in statistical software is not just a trend; it's a transformation that enhances decision-making capabilities in business.
Cloud-Based Solutions
Cloud-based statistical software is another trend that has gained momentum. The cloud offers flexibility and scalability, which are essential for businesses that process large amounts of data. Unlike traditional software that requires significant IT resources for maintenance, cloud solutions can be accessed securely from anywhere with an internet connection.
For small to medium-sized businesses, cloud-based tools provide cost-effective solutions without the need for heavy upfront investments. Subscription models allow users to pay for only what they need. This approach can allocate resources more efficiently, making statistical analysis accessible to organizations of varying sizes.
Additionally, cloud solutions facilitate collaboration. Teams working remotely can analyze and share data seamlessly. This enhances workflow efficiency and encourages a shared environment for decision-making that is informed by comprehensive analytics.
End
The conclusion serves as a critical part of this article, tying together the discussions on statistical software while emphasizing its relevance for small to medium-sized businesses, entrepreneurs, and IT professionals. In the ever-evolving landscape of data analysis, the need for effective statistical software is of utmost importance. Choosing the right software can greatly influence the outcomes of analysis and decision-making processes.
Making an Informed Choice
When it comes to making an informed choice regarding statistical software, several factors must be taken into consideration. First, understanding oneβs own specific needs is crucial. This includes identifying the types of data that will be analyzed and the statistical methods required. Some software is tailored for specific tasks, while others offer a broader range of functionalities.
Key Elements to Consider:
- User level: Consider whether the software is suitable for beginners or more advanced users.
- Features: Look for software that provides the necessary statistical techniques relevant to your analysis.
- Technical support: Reliable support can save time and frustration.
- Community and resources: A strong user community can be beneficial for learning and troubleshooting.
- Cost: Evaluate whether the software fits your budget, especially if you are a small business.
By examining these factors, one can increase the likelihood of selecting software that enhances productivity and supports data-driven decision-making.
The Importance of Compatibility
Compatibility is another vital consideration when selecting statistical software. As businesses expand and technological landscapes change, the need for seamless integration with existing tools becomes apparent. Many firms use various digital environments, making it essential that statistical software can connect with different databases, data visualization tools, or data management systems.
Benefits of Compatibility:
- Improved Data Flow: Compatibility allows for smoother data transfer between systems.
- Reduced Learning Curve: Familiar interfaces can reduce training times for teams.
- Greater Flexibility: Adaptability to new tools and technologies can enhance analytical capabilities.
In summary, both making informed choices and ensuring compatibility are significant elements characterized in the decision-making process surrounding statistical software. Such considerations not only foster an efficient analytical environment but also provide an edge in deriving actionable insights.